- June 19~24, 2022
- New Orleans, Louisiana
- Computer Vision
CVPR 2022
IEEE Conference on Computer Vision and Pattern Recognition (CVPR) 2022
The IEEE / CVF Computer Vision and Pattern Recognition Conference (CVPR) is the premier annual computer vision event comprising the main conference and several co-located workshops and short courses. Sony will exhibit and participate as a Gold sponsor.
Recruit information for CVPR-2022
We look forward to highly motivated individuals applying to Sony so that we can work together to fill the world with emotion and pioneer the future with dreams and curiosity. Join us and be part of a diverse, innovative, creative, and original team to inspire the world.
For Sony AI positions, please see https://ai.sony/joinus/jobroles/.
*The special job offer for CVPR-2022 has closed. Thank you for many applications.
Technologies & Workshops
Technology 01 On-the-fly 6DoF object pose estimation
For applications involving interaction with the real world, such as AR, 3 dimensional(6DoF) pose estimation is a key technology. However, modern ML methods require a large amount of labeling effort, which prevents users to improvise and register their favorites.
Our technology solves this issue while achieving a high level of accuracy.
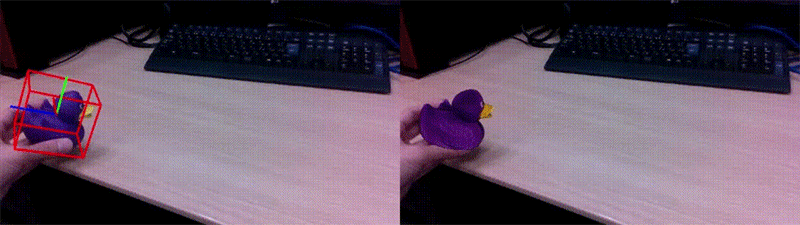
In the right part of the clip above, a 3D model of the object is rendered based on the pose estimation results.
Pick up any object you like and turn it around in front of the camera. This is all it takes to register it.
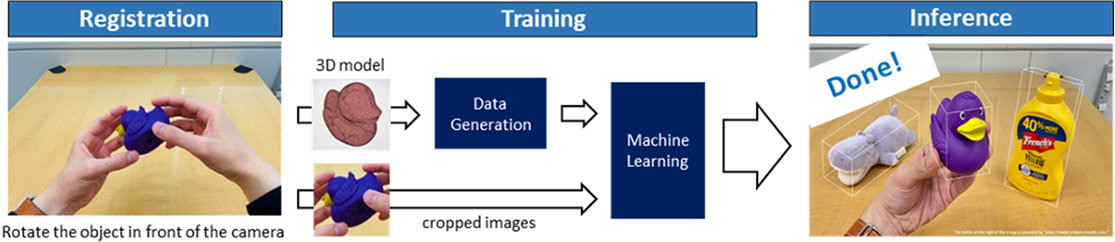
Technology 02 Lensless Imaging Technology
What is a lensless camera?
A lensless camera is a camera consisting of an image sensor and a patterned mask. In contrast to a standard camera with a lens, a sensor image of a lensless camera does not resemble the scene image and should be decoded. For example, in Fig. 1, we show a basic imaging pipeline for a lensless camera. A lensless camera can be an attractive alternative to a lens-based camera despite the necessary image reconstruction step. As one of the largest image sensors and sensing electronics manufacturers, Sony is developing lensless imaging technology with a perspective of applying it in our future products.
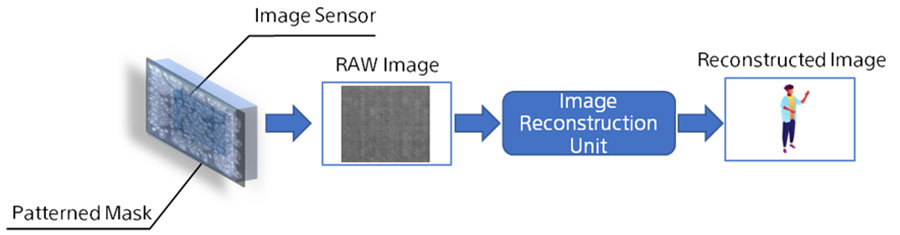
Fig. 1. Lensless imaging pipeline
Lensless imaging in long-wave infrared
One advantage of a lensless camera is that it can be attractive when light focusing with a lens is impossible or too expensive. One such example is long-wave infrared imaging (LWIR), where one of the biggest challenges is the high price of optics. In our recent project, we successfully constructed an LWIR lensless camera and demonstrated how it could be used in combination with a conventional RGB camera to improve the accuracy of pedestrian detection in challenging illumination scenarios. In Fig. 2, we show a schematic of the image processing pipeline. See [1] for more details.
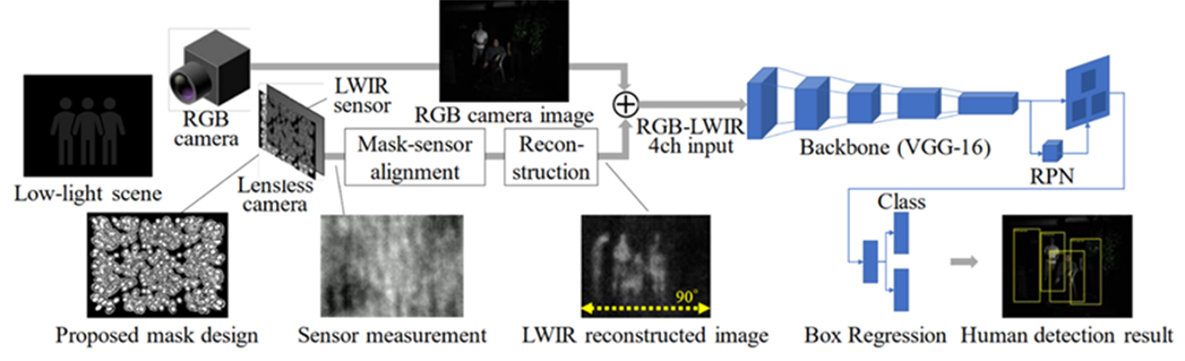
Fig. 2. LWIR lensless + RGB imaging pipeline
Lensless mismatched aspect ratio imaging
Lensless imaging technology can also be used to achieve results that are impossible with a regular camera. Recently, we demonstrated a lensless camera for imaging with a resolution significantly larger than the sensor's resolution in one of its dimensions by virtually rearranging the available pixels. For example, in Fig. 3, we demonstrate the reconstruction result from our lensless camera, which allows us to capture scene images with a resolution of 3171 x 103 pixels from a 453 x 721 sensor image. I.e., the horizontal resolution of the result is more than four times higher than the vertical resolution of the sensor. For more details, see [2].

Fig. 3. Sensor image (left), reconstruction result (right)
Efficient optical implementation for lensless technology
In a lensless camera, a patterned mask is often the only optical element between the scene and the sensor. Therefore, it is important to ensure that the mask works with maximum efficiency. Typically, many lensless cameras employ binary or phase masks. However, these masks have several issues, including low light efficiency and diffraction-related artifacts. Recently, we have proposed a framework for migration from diffractive to refractive masks, which allows us to significantly improve imaging quality without adding extra computational or manufacturing costs. Fig. 4 shows the reconstruction quality improvement by a simple switching from a binary to a refractive mask. For more details, see [3].
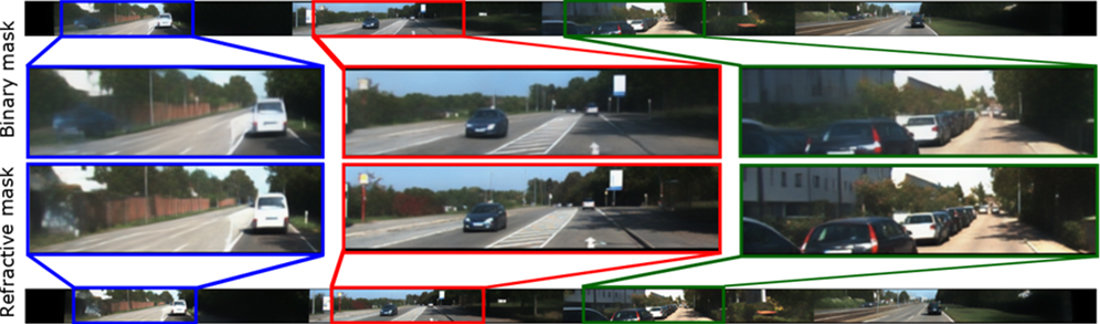
Fig. 4. Reconstruction results using a binary mask (top) and a refractive mask (bottom)
[1] I. Reshetouski, H. Oyaizu, K. Nakamura, R. Satoh, S. Ushiki, R. Tadano, A. Ito, and J. Murayama, "Lensless Imaging with Focusing Sparse URA Masks in Long-Wave Infrared and its Application for Human Detection,“ 2020 European Conference on Computer Vision (ECCV)
[2] I. Reshetouski, R. Tadano, H. Oyaizu, K. Nakamura and J. Murayama, "Lensless Mismatched Aspect Ratio Imaging," 2021 IEEE International Conference on Computational Photography (ICCP), 2021, pp. 1-12
[3] To appear in 2022 Imaging and Applied Optics Congress
Technology 03 Polarization Image Sensor Technology Polarsens
What is Polarization? What is Polarsens? Why does an on chip Polarizer have different filter angles?
This video provides a general overview of Sony Semiconductor Solutions' Polarization Image Sensor.
This video introduces application examples of polarization image sensor: reflection removal, visual inspection, improve visibility, 3D/Stress detection and human/vehicle detection.
Technology 05 AR Gaming with RGB / Depthsense enabled SLAM
Integration of RGB-D Fusion and SLAM into a real-time Mobile AR Demo Application. Capitalizing on Time of Flight for instant SLAM/3D, low-light/texture SLAM/3D, night vision and scene mesh. Dense depth (full-field) and sparse depth (spots) based pipelines.
Workshop 01 A Low Memory Footprint Quantized Neural Network for Depth Completion of Very Sparse Time-off-Flight Depth Maps
Sparse active illumination enables precise time-of-flight depth sensing as it maximizes signal-to-noise ratio for low power budgets. However, depth completion is required to produce dense depth maps for 3D perception. We address this task with realistic illumination and sensor resolution constraints by simulating ToF datasets for indoor 3D perception with challenging sparsity levels. We propose a quantized convolutional encoder-decoder network for this task. Our model achieves optimal depth map quality by means of input pre-processing and carefully tuned training with a geometry-preserving loss function. We also achieve low memory footprint for weights and activations by means of mixed precision quantization-at-training techniques. The resulting quantized models are comparable to the state of the art in terms of quality, but they require very low GPU
times and achieve up to 14-fold memory size reduction for the weights w.r.t. their floating point counterpart with minimal impact on quality metrics.
This is a joint work between Sony Depthsensing Solutions, Sony R&D Center Stuttgart Lab 1, and the University of Padua, Italy.
Workshop 02 M2FNet: Multi-modal Fusion Network for Emotion Recognition in Conversation
Emotion Recognition in Conversations (ERC) is crucial in developing sympathetic human-machine interaction. In conversational videos, emotion can be present in audio, visual, and transcript.
However, due to the inherent characteristics of these modalities, multi-modal ERC has always been considered a challenging undertaking. This paper proposes a Multi-modal Fusion Network (M2FNet) that extracts emotion-relevant features from visual, audio, and text modality. It further employs a multi-head attention-based fusion mechanism to combine emotion-rich latent representations of the input data.
A new feature extractor is also introduced to extract features from audio and visual modalities. The proposed M2FNet architecture outperforms the other methods and sets a new state-of-the-art performance.
Workshop 03 SqueezeNeRF: Further factorized FastNeRF for memory-efficient inference
Neural Radiance Fields (NeRF) has emerged as the state-of-the-art method for novel view generation of complex scenes, but is very slow during inference.
Recently, there have been multiple works on speeding up NeRF inference, but the state of the art methods for real-time NeRF inference rely on caching the neural network output, which occupies several giga-bytes of disk space that limits their applicability in embedded systems. In this work, we propose SqueezeNeRF, which is more than 60 times memory-efficient than the state of the art methods and is still able to render at more than 190 frames per second on a high spec GPU during inference.
Publications
Publication 01: CVPR Degree-of-linear-polarization-based Color Constancy
- Authors
- Taishi Ono Yuhi Kondo, Legong Sun, Teppei Kurita, Yusuke Moriuchi (Sony Group Corporation R&D Center)
- Abstract
- Color constancy is an essential function in digital photography and a fundamental process for many computer vision applications. Accordingly, many methods have been proposed, and some recent ones have used deep neural networks to handle more complex scenarios. However, both the traditional and latest methods still impose strict assumptions on their target scenes in explicit or implicit ways. This paper shows that the degree of linear polarization dramatically solves the color constancy problem because it allows us to find achromatic pixels stably. Because we only rely on the physics-based polarization model, we significantly reduce the assumptions compared to existing methods. Furthermore, we captured a wide variety of scenes with ground-truth illuminations for evaluation, and the proposed approach achieved state-of-the-art performance with a low computational cost. Additionally, the proposed method can estimate illumination colors from chromatic pixels and manage multi-illumination scenes. Lastly, the evaluation scenes and codes are publicly available to encourage more development in this field.
Publication 02: CVPR Workshop "5th Efficient Deep Learning for Computer Vision" A Low Memory Footprint Quantized Neural Network for Depth Completion of Very Sparse Time-of-Flight Depth Maps
- Authors
- Xiaowen Jiang, Valerio Cambareri, Gianluca Agresti, Cynthia Ugwu, Adriano Simonetto, Fabien Cardinaux and Pietro Zanuttigh (EPFL, Sony Depthsensing Solutions NV, Sony Europe B.V. R&D Center Stuttgart Laboratory 1, University of Padova)
- Abstract
- Sparse active illumination enables precise time-of-flight depth sensing as it maximizes signal-to-noise ratio for low power budgets. However, depth completion is required to produce dense depth maps for 3D perception. We address this task with realistic illumination and sensor resolution constraints by simulating ToF datasets for indoor 3D perception with challenging sparsity levels. We propose a quantized convolutional encoder-decoder network for this task. Our model achieves optimal depth map quality by means of input pre-processing and carefully tuned training with a geometry-preserving loss function. We also achieve low memory footprint for weights and activations by means of mixed precision quantization-at-training techniques. The resulting quantized models are comparable to the state of the art in terms of quality, but they require very low GPU times and achieve up to 14-fold memory size reduction for the weights w.r.t. their floating point counterpart with minimal impact on quality metrics.
Publication 03: CVPR Workshop "5th Multimodal Learning and Applications" M2FNet: Multi-Modal Fusion Network for Emotion Recognition in Conversation
- Authors
- Vishal Chudasama, Purbayan Kar, Ashish Gudmalwar, Nirmesh Shah, Pankaj Wasnik, Naoyuki Onoe (Sony Research India)
- Abstract
- Emotion Recognition in Conversations (ERC) is crucial in developing sympathetic human-machine interaction. In conversational videos, emotion can be present in multiplemodalities, i.e., audio, video, and transcript. However, due to the inherent characteristics of these modalities, multi-modal ERC has always been considered a challenging undertaking.
Existing ERC research focuses mainly on using text information in a discussion, ignoring the other two modalities. We anticipate that emotion recognition accuracy can be improved by employing a multi-modal approach.
Thus, in this study, we propose a Multi-modal Fusion Network (M2FNet) that extracts emotion-relevant features from visual, audio, and text modality. It employs a multi-head attention-based fusion mechanism to combine emotion-rich latent representations of the input data. We introduce a new feature extractor to extract latent features from the audio and visual modality. The proposed feature extractor is trained with a novel adaptive marginbased triplet loss function to learn emotion-relevant features from the audio and visual data. In the domain of ERC, the existing methods perform well on one benchmark dataset but not on others. Our results show that the proposed M2FNet architecture outperforms all other methods in terms of weighted average F1 score on well-known MELD and IEMOCAP datasets and sets a new state-of-theart performance in ERC.
Publication 04: CVPR Workshop "Efficient Deep Learning for Computer Vision" SqueezeNeRF: Further factorized FastNeRF for memory-efficient inference
- Authors
- Krishna Wadhwani, Tamaki Kojimma (Sony Group Corporation R&D Center)
- Abstract
- Neural Radiance Fields (NeRF) has emerged as the state-of-the-art method for novel view generation of complex scenes, but is very slow during inference. Recently, there have been multiple works on speeding up NeRF inference, but the state of the art methods for real-time NeRF inference rely on caching the neural network output, which occupies several giga-bytes of disk space that limits their real-world applicability. As caching the neural network of original NeRF network is not feasible, Garbin et al. proposed "FastNeRF" which factorizes the problem into 2 sub-networks - one which depends only on the 3D coordinate of a sample point and one which depends only on the 2D camera viewing direction. Although this factorization enables them to reduce the cache size and perform inference at over 200 frames per second, the memory overhead is still substantial. In this work, we propose SqueezeNeRF, which is more than 60 times memory-efficient than the sparse cache of FastNeRF and is still able to render at more than 190 frames per second on a high spec GPU during inference.
Publication 05: CVPR Workshop "Learning with Limited Labelled Data for Image and Video Understanding" Bootstrapped Representation Learning for Skeleton-Based Action Recognition
- Authors
- Olivier Moliner, Sangxia Huang, Kalle Åström (Lund University, Sony Europe B.V. R&D Center Lund Laboratory)
- Abstract
- In this work, we study self-supervised representation learning for 3D skeleton-based action recognition. We extend Bootstrap Your Own Latent (BYOL) for representation learning on skeleton sequence data and propose a new data augmentation strategy including two asymmetric transformation pipelines. We also introduce a multi-viewpoint sampling method that leverages multiple viewing angles of the same action captured by different cameras. In the semisupervised setting, we show that the performance can be further improved by knowledge distillation from wider networks, leveraging once more the unlabeled samples. We conduct extensive experiments on the NTU-60, NTU-120 and PKU-MMD datasets to demonstrate the performance of our proposed method. Our method consistently outperforms the current state of the art on linear evaluation, semisupervised and transfer learning benchmarks.
Other Conferences
-
- May 7 ~ 13, 2022 /May 22 ~ 27, 2022
- Online / Singapore, China
- Signal Processing
ICASSP2022
2022 IEEE International Conference on Acoustics, Speech and Signal Processing
View our activities -
- February 22~ March 1, 2022
- Online
- ML/General AI
AAAI-2022
The Thirty-Sixth AAAI Conference on Artificial Intelligence
View our activities