- May 7 ~ 13, 2022 /May 22 ~ 27, 2022
- Online / Singapore, China
- Signal Processing
ICASSP2022
2022 IEEE International Conference on Acoustics, Speech and Signal Processing
The International Conference on Acoustics, Speech, & Signal Processing (ICASSP), is the IEEE Signal Processing Society’s flagship conference on signal processing and its applications. The 47th edition of ICASSP will be held in Singapore. The program will include keynotes by pre-eminent international speakers, cutting-edge tutorial topics, and forward-looking special sessions.
Recruit information for ICASSP-2022
We look forward to highly motivated individuals applying to Sony so that we can work together to fill the world with emotion and pioneer the future with dreams and curiosity. Join us and be part of a diverse, innovative, creative, and original team to inspire the world.
For Sony AI positions, please see https://ai.sony/joinus/jobroles/.
*The special job offer for ICASSP-2022 has closed. Thank you for many applications.
Technologies & Business use case
Theme 01 360 Reality Audio
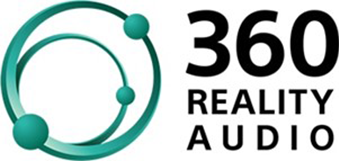
360 Reality Audio is a new music experience that uses Sony's object-based 360 Spatial Sound technologies. Individual sounds such as vocals, chorus, piano, guitar, bass and even sounds of the live audience can be placed in a 360 spherical sound field, giving artists and creators a new way to express their creativity. Listeners can be immersed in a field of sound exactly as intended by artists and creators.
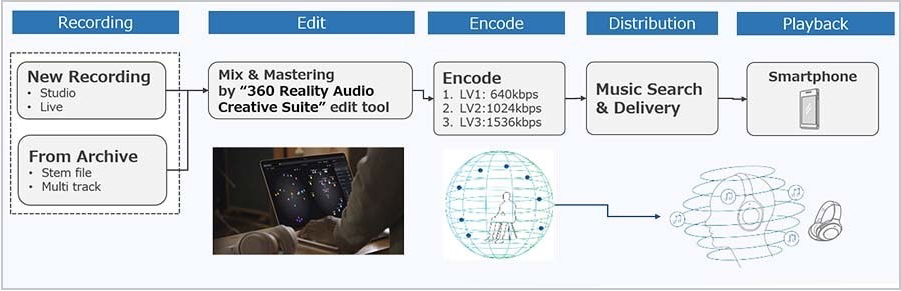
360 Spatial Sound Personalization for more immersive 360 Reality Audio experience
Optimization by personal ear data uses Sony's original estimation algorithm utilizing machine learning. We analyze the listener's hearing characteristics by estimating the 3D shape of the ear based a photo of their ear through "Sony | Headphones Connect" app.
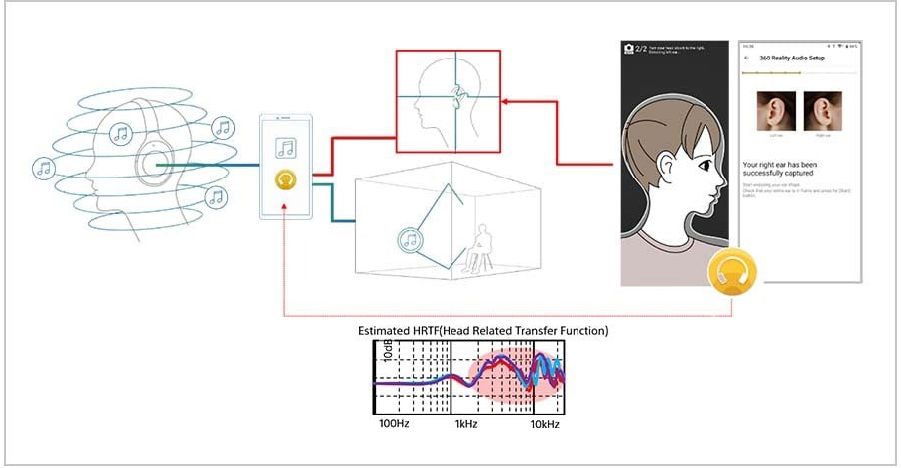
Theme 02 Speak to Chat
"Speak-to-Chat" UX Overview :
Speak-to-Chat allows you to speak to others while wearing your headphone. When you speak to others, AI detects your speech and changes mode for be able to conversation.
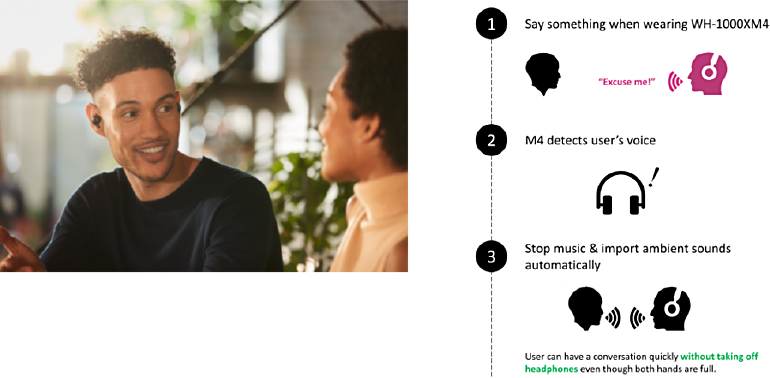
"Speak-to-Chat" Algorithm :
"Speak-to-Chat" is realized by Sony's unique technologies. Precise Voice Pickup Technology captures user's voice even in noisy situations. Speech Detect Algorithm is created by using machine learning technology.
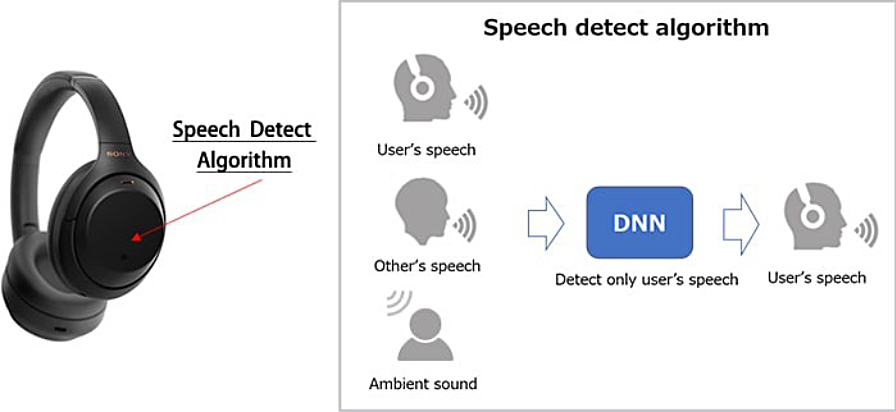
Theme 03 Character Conversation AI technology
People wish to talk freely with their favorite characters, create AI characters on their own and provide various services using AI characters.
At the Sony Group R&D Center, we are working on the character conversation AI technology to make these dreams come true. Interacting with characters in a more realistic way requires not just natural language processing technology for language understanding and generation but many other underlying technologies as well, including voice signal processing technology for voice recognition and speech synthesis and image processing technology for image recognition and graphic expression.
Deliver exciting experiences by combining the technologies to turn creators’ imaginations into reality with the Sony Group’s entertainment prowess - that’s what we are developing the character conversation AI technology for. In the context of meeting this goal, we are proposing new values using this technology through open demonstration experiments and application development activities.
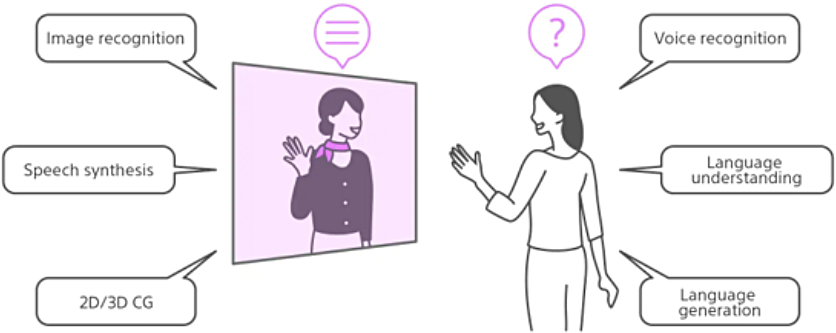
Theme 04 Business case study and open experiment of the character conversation AI technology
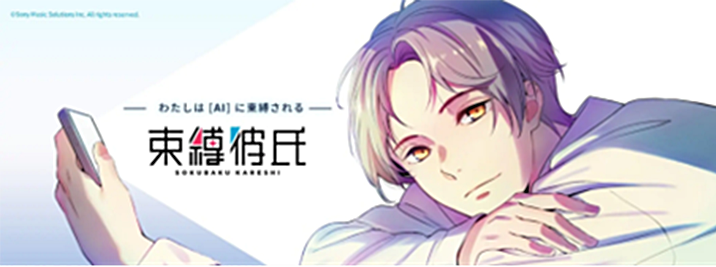
Character of SOKUBAKU KARESHI - Aki Shindo ©Sony Music Solutions Inc. All rights reserved.
SWORD ART ONLINE EX-CHRONICLE - AI conversation experiment with Takeru Higa on AI creation and personality development
©2020 REKI KAWAHARA/KADOKAWA CORPORATION/SAO-P Project
©BANDAI NAMCO Entertainment Inc.
A CG model from SWORD ART ONLINE Alicization Lycoris, a home game from BANDAI NAMCO Entertainment Inc., was used.
Participants posted their desired conversations via the Web in advance, and these conversations were used to create AI that reflected the personality of each individual participant. At the event, the conversations between the AI of participants and Alice were demonstrated according to the world view of SWORD ART ONLINE. The participants saw AI speak just like they do, even on topics their posts did not mention, making them feel as if they were in the world of SWORD ART ONLINE.
Contributions to Research Challenges
Challenge 01 No.1 in Task 3 of DCASE2021
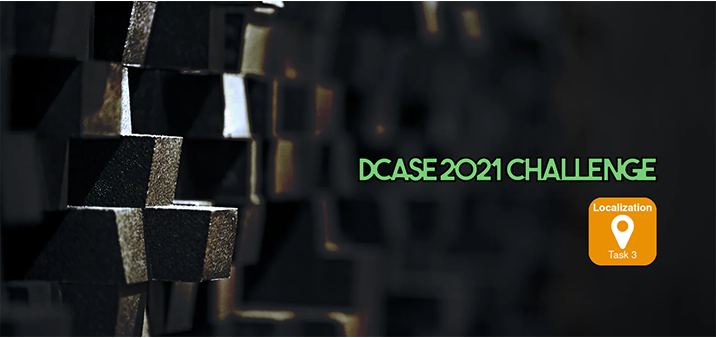
Sony has won the first place in Task 3 of DCASE 2021, the world's largest international competition for the detection and classification of acoustic scenes and events (https://dcase.community/challenge2021/task-sound-event-localization-and-detection-results). The goal of Task 3 "Sound Event Localization and Detection with Directional Interference" is to recognize individual sound events of specific classes by detecting their temporal activity and estimating their location in the presence of spatial ambient noise and interfering directional events that do not belong to the target classes.
Our system is based on the activity-coupled Cartesian direction of arrival (ACCDOA) representation that enables us to solve a SELD task with a single target. Using the ACCDOA-based system with RD3Net as the backbone, we realize model ensembles. We further propose impulse response simulation (IRS), which generates simulated multi-channel signals by convolving simulated room impulse responses (RIRs) with source signals extracted from the original dataset.
ACCDOA
Kazuki Shimada, Yuichiro Koyama, Naoya Takahashi, Shusuke Takahashi, and Yuki Mitsufuji, "ACCDOA: Activity-coupled cartesian direction of arrival representation for sound event localization and detection," in Proc. of IEEE ICASSP, 2021.
RD3Net
Naoya Takahashi and Yuki Mitsufuji, "Densely connected multidilated convolutional networks for dense prediction tasks," in Proc. of IEEE CVPR, 2021.
IRS
Yuichiro Koyama, Kazuhide Shigemi, Masafumi Takahashi, Kazuki Shimada, Naoya Takahashi, Emiru Tsunoo, Shusuke Takahashi, Yuki Mitsufuji, "Spatial data augmentation with simulated room impulse responses for sound event localization and detection," in Proc. of IEEE ICASSP, 2022.
Challenge 02 Organizer of Task 3 in DCASE2022 Challenge
Sony is organizing Task 3 of the DCASE2022 Challenge "Sound Event Localization and Detection Evaluated in Real Spatial Sound Scenes" together with the Audio Research Group (ARG) of Tampere University, where the submitted SELD systems will be evaluated on recordings from real sound scenes.
For this task, we created Sony-TAu Realistic Spatial Soundscapes 2022 (STARSS22), a new dataset which consists of multichannel recordings of real scenes in various room environments along with annotations for spatial and temporal occurrences of target sound events.
A publicly available implementation of our innovative training scheme called MULTI-ACCDOA is available on GitHub and is provided as baseline system for Task 3.
MULTI-ACCDOA
MULTI-ACCDOA
Kazuki Shimada, Yuichiro Koyama, Shusuke Takahashi, Naoya Takahashi, Emiru Tsunoo, Yuki Mitsufuji, "Multi-ACCDOA: Localizing and Detecting Overlapping Sounds from the Same Class with Auxiliary Duplicating Permutation Invariant Training," in Proc. of IEEE ICASSP, 2022.
Challenge 03 Music Demixing Challenge 2021
AIcrowd Competition – We organized an online competition for research and industry (https://www.aicrowd.com/challenges/music-demixing-challenge-ismir-2021). For this competition, a new dataset from SMEJ was created, which was not accessible to the participants and ensured a fair evaluation of the system. The competition was well received by the community, getting in total 1,541 submissions from 41 teams around the world. During the competition, we hosted online meetings with the participants to discuss ideas and problems related to audio source separation and, more generally, audio signal processing.
Conference Workshop – After the end of the challenge, we organized a workshop (https://mdx-workshop.github.io) on audio source separation in parallel with the ISMIR 2021 conference: of the 18 proposals we received, the workshop featured 9 presentations, one keynote and one symposium, all including prominent figures in the academic and industry. The workshop got a lot of interest with 162 attendants.
Journal Paper – In order to share the lessons that we learned in organizing this challenge, we organized a joint writing effort and prepared a scientific paper together with the winning teams: Y. Mitsufuji, G. Fabbro, S. Uhlich, F.-R. Stöter, A. Défossez, M. Kim, W. Choi, C.-Y. Yu, K.-W. Cheuk: Music Demixing Challenge 2021, Frontiers in Signal Processing, 2022 (https://doi.org/10.3389/frsip.2021.808395)
Publications
Publication 01 Amicable Examples for Informed Source Separation
- Authors
- Naoya Takahashi, Yuki Mitsufuji
- Abstract
- This paper deals with the problem of informed source separation (ISS), where the sources are accessible during the so-called encoding stage. Previous works computed side-information during the encoding stage and source separation models were designed to utilize the side-information to improve the separation performance. In contrast, in this work, we improve the performance of a pretrained separation model that does not use any side-information. To this end, we propose to adopt an adversarial attack for the opposite purpose, i.e., rather than computing the perturbation to degrade the separation, we compute an imperceptible perturbation called amicable noise to improve the separation. Experimental results show that the proposed approach selectively improves the performance of the targeted separation model by 2.23 dB on average and is robust to signal compression. Moreover, we propose multi-model multi-purpose learning that control the effect of the perturbation on different models individually.
Publication 02 Source Mixing and Separation Robust Audio Steganography
- Authors
- Naoya Takahashi, Mayank Kumar Singh, Yuki Mitsufuji
- Abstract
- Audio steganography aims at concealing secret information in carrier audio with imperceptible modification on the carrier. Although previous works addressed the robustness of concealed message recovery against distortions introduced during transmission, they do not address the robustness against aggressive editing such as mixing of other audio sources and source separation. In this work, we propose for the first time a steganography method that can embed information into individual sound sources in a mixture such as instrumental tracks in music. To this end, we propose a time-domain model and curriculum learning essential to learn to decode the concealed message from the separated sources. Experimental results show that the proposed method successfully conceals the information in an imperceptible perturbation and that the information can be correctly recovered even after mixing of other sources and separation by a source separation algorithm. Furthermore, we show that the proposed method can be applied to multiple sources simultaneously without interfering with the decoder for other sources even after the sources are mixed and separated.
Publication 03 Automatic DJ Transitions with Differentiable Audio Effects and Generative Adversarial Networks
- Authors
- Bo-Yu Chen, Wei-Han Hsu, Wei-Hsiang Liao, Marco A. Martínez Ramírez, Yuki Mitsufuji, Yi-Hsuan Yang
- Abstract
- A central task of a Disc Jockey (DJ) is to create a mix set of music with seamless transitions between adjacent tracks. In this paper, we explore a data-driven approach that uses a generative adversarial network to create the song transition by learning from real-world DJ mixes. In particular, the generator of the model uses two differentiable digital signal processing components, an equalizer (EQ) and a fader, to mix two tracks selected by a data generation pipeline. The generator has to set the parameters of the EQs and fader in such a way that the resulting mix resembles real mixes created by human DJ, as judged by the discriminator counterpart. Result of a listening test shows that the model can achieve competitive results compared with a number of baselines.
Publication 04 Music Source Separation with Deep Equilibrium Models
- Authors
- Yuichiro Koyama, Naoki Murata, Stefan Uhlich, Giorgio Fabbro, Shusuke Takahashi, Yuki Mitsufuji
- Abstract
- While deep neural network-based music source separation (MSS) is very effective and achieves high performance, its model size is often a problem for practical deployment. Deep implicit architectures such as deep equilibrium models (DEQ) were recently proposed, which can achieve higher performance than their explicit counterparts with limited depth while keeping the number of parameters small. This makes DEQ also attractive for MSS, especially as it was originally applied to sequential modeling tasks in natural language processing and thus should in principle be also suited for MSS. However, an investigation of a good architecture and training scheme for MSS with DEQ is needed as the characteristics of acoustic signals are different from those of natural language data. Hence, in this paper we propose an architecture and training scheme for MSS with DEQ. Starting with the architecture of Open-Unmix (UMX), we replace its sequence model with DEQ. We refer to our proposed method as DEQ-based UMX (DEQ-UMX). Experimental results show that DEQ-UMX performs better than the original UMX while reducing its number of parameters by 30%.
Publication 05 Spatial Data Augmentation with Simulated Room Impulse Responses for Sound Event Localization and Detection
- Authors
- Yuichiro Koyama, Kazuhide Shigemi, Masafumi Takahashi, Kazuki Shimada, Naoya Takahashi, Emiru Tsunoo, Shusuke Takahashi, Yuki Mitsufuji
- Abstract
- Recording and annotating real sound events for a sound event localization and detection (SELD) task is time consuming, and data augmentation techniques are often favored when the amount of data is limited. However, how to augment the spatial information in a dataset, including unlabeled directional interference events, remains an open research question. Furthermore, directional interference events make it difficult to accurately extract spatial characteristics from target sound events. To address this problem, we propose an impulse response simulation framework (IRS) that augments spatial characteristics using simulated room impulse responses (RIR). RIRs corresponding to a microphone array assumed to be placed in various rooms are accurately simulated, and the source signals of the target sound events are extracted from a mixture. The simulated RIRs are then convolved with the extracted source signals to obtain an augmented multi-channel training dataset. Evaluation results obtained using the TAU-NIGENS Spatial Sound Events 2021 dataset show that the IRS contributes to improving the overall SELD performance. Additionally, we conducted an ablation study to discuss the contribution and need for each component within the IRS.
Publication 06 Multi-ACCDOA: Localizing and Detecting Overlapping Sounds from the Same Class with Auxiliary Duplicating Permutation Invariant Training
- Authors
- Kazuki Shimada, Yuichiro Koyama, Shusuke Takahashi, Naoya Takahashi, Emiru Tsunoo, Yuki Mitsufuji
- Abstract
- Sound event localization and detection (SELD) involves identifying the direction-of-arrival (DOA) and the event class. The SELD methods with a class-wise output format make the model predict activities of all sound event classes and corresponding locations. The class-wise methods can output activity-coupled Cartesian DOA (ACCDOA) vectors, which enable us to solve a SELD task with a single target using a single network. However, there is still a challenge in detecting the same event class from multiple locations. To overcome this problem while maintaining the advantages of the class-wise format, we extended ACCDOA to a multi one and proposed auxiliary duplicating permutation invariant training (ADPIT). The multi- ACCDOA format (a class- and track-wise output format) enables the model to solve the cases with overlaps from the same class. The class-wise ADPIT scheme enables each track of the multi-ACCDOA format to learn with the same target as the single-ACCDOA format. In evaluations with the DCASE 2021 Task 3 dataset, the model trained with the multi-ACCDOA format and with the class-wise ADPIT detects overlapping events from the same class while maintaining its performance in the other cases. Also, the proposed method performed comparably to state-of-the-art SELD methods with fewer parameters.
Publication 07 Spatial Mixup: Directional Loudness Modification as Data Augmentation for Sound Event Localization and Detection
- Authors
- Ricardo Falcon-Perez, Kazuki Shimada, Yuichiro Koyama, Shusuke Takahashi, Yuki Mitsufuji
- Abstract
- Data augmentation methods have shown great importance in diverse supervised learning problems where labeled data is scarce or costly to obtain. For sound event localization and detection (SELD) tasks several augmentation methods have been proposed, with most borrowing ideas from other domains such as images, speech, or monophonic audio. However, only a few exploit the spatial properties of a full 3D audio scene. We propose Spatial Mixup, as an application of parametric spatial audio effects for data augmentation, which modifies the directional properties of a multi-channel spatial audio signal encoded in the ambisonics domain. Similarly to beamforming, these modifications enhance or suppress signals arriving from certain directions, although the effect is less pronounced. Therefore enabling deep learning models to achieve invariance to small spatial perturbations. The method is evaluated with experiments in the DCASE 2021 Task 3 dataset, where spatial mixup increases performance over a non-augmented baseline, and compares to other well known augmentation methods. Furthermore, combining spatial mixup with other methods greatly improves performance.
Publication 08 Improving Character Error Rate Is Not Equal to Having Clean Speech: Speech Enhancement for ASR Systems with Black-box Acoustic Models
- Authors
- Ryosuke Sawata, Yosuke Kashiwagi, Shusuke Takahashi
- Abstract
- A deep neural network (DNN)-based speech enhancement (SE) aiming to maximize the performance of an automatic speech recognition (ASR) system is proposed in this paper. In order to optimize the DNN-based SE model in terms of the character error rate (CER), which is one of the metric to evaluate the ASR system and generally non-differentiable, our method uses two DNNs: one for speech processing and one for mimicking the output CERs derived through an acoustic model (AM). Then both of DNNs are alternately optimized in the training phase. Even if the AM is a black-box, e.g., like one provided by a third-party, the proposed method enables the DNN-based SE model to be optimized in terms of the CER since the DNN mimicking the AM is differentiable. Consequently, it becomes feasible to build CER-centric SE model that has no negative effect, e.g., additional calculation cost and changing network architecture, on the inference phase since our method is merely a training scheme for the existing DNN-based methods. Experimental results show that our method improved CER by 8.8% relative derived through a black-box AM although certain noise levels are kept.
Publication 09 Run-And-Back Stitch Search: Novel Block Synchronous Decoding for Streaming Encoder-Decoder ASR
- Authors
- Emiru Tsunoo, Chaitanya Narisetty, Michael Hentschel, Yosuke Kashiwagi, Shinji Watanabe
- Abstract
- A streaming style inference of encoder-decoder automatic speech recognition (ASR) system is important for reducing latency, which is essential for interactive use cases. To this end, we propose a novel blockwise synchronous decoding algorithm with a hybrid approach that combines endpoint prediction and endpoint post-determination. In the endpoint prediction, we compute the expectation of the number of tokens that are yet to be emitted in the encoder features of the current blocks using the CTC posterior. Based on the expectation value, the decoder predicts the endpoint to realize continuous block synchronization, as a running stitch. Meanwhile, endpoint post-determination probabilistically detects backward jump of the source-target attention, which is caused by the misprediction of endpoints. Then it resumes decoding by discarding those hypotheses, as back stitch. We combine these methods into a hybrid approach, namely run-and-back stitch search, which reduces the computational cost and latency. Evaluations of various ASR tasks show the efficiency of our proposed decoding algorithm, which achieves a latency reduction, for instance in the Librispeech test set from 1487 ms to 821 ms at the 90th percentile, while maintaining a high recognition accuracy.
Publication 10 Joint Speech Recognition and Audio Captioning
- Authors
- Chaitanya Narisetty, Emiru Tsunoo, Xuankai Chang, Yosuke Kashiwagi, Michael Hentschel, Shinji Watanabe
- Abstract
- Speech samples recorded in both indoor and outdoor environments are often contaminated with secondary audio sources. Most end-to-end monaural speech recognition systems either remove these background sounds using speech enhancement or train noise-robust models. For better model interpretability and holistic understanding, we aim to bring together the growing field of automated audio captioning (AAC) and the thoroughly studied automatic speech recognition (ASR). The goal of AAC is to generate natural language descriptions of contents in audio samples. We propose several approaches for end-to-end joint modeling of ASR and AAC tasks and demonstrate their advantages over traditional approaches, which model these tasks independently. A major hurdle in evaluating our proposed approach is the lack of labeled audio datasets with both speech transcriptions and audio captions. Therefore we also create a multi-task dataset by mixing the clean speech Wall Street Journal corpus with multiple levels of background noises chosen from the AudioCaps dataset. We also perform extensive experimental evaluation and show improvements of our proposed methods as compared to existing state-of-the-art ASR and AAC methods.
Publication 11 Polyphone Disambiguation and Accent Prediction Using Pre-trained Language Models in Japanese TTS Front-End
- Authors
- Rem Hida, Masaki Hamada, Chie Kamada, Emiru Tsunoo, Toshiyuki Sekiya, Toshiyuki Kumakura
- Abstract
- Although end-to-end text-to-speech (TTS) models can generate natural speech, challenges still remain when it comes to estimating sentence-level phonetic and prosodic information from raw text in Japanese TTS systems. In this paper, we propose a method for polyphone disambiguation (PD) and accent prediction (AP). The proposed method incorporates explicit features extracted from morphological analysis and implicit features extracted from pre-trained language models (PLMs). We use BERT and Flair embeddings as implicit features and examine how to combine them with explicit features. Our objective evaluation results showed that the proposed method improved the accuracy by 5.7 points in PD and 6.0 points in AP. Moreover, the perceptual listening test results confirmed that a TTS system employing our proposed model as a front-end achieved a mean opinion score close to that of synthesized speech with ground-truth pronunciation and accent in terms of naturalness.
Publication 12 NVC-Net: End-to-End Adversarial Voice Conversion
- Authors
- Bac Nguyen, Fabien Cardinaux
- Abstract
- Voice conversion has gained increasing popularity in many applications of speech synthesis. The idea is to change the voice identity from one speaker into another while keeping the linguistic content unchanged. Many voice conversion approaches rely on the use of a vocoder to reconstruct the speech from acoustic features, and as a consequence, the speech quality heavily depends on such a vocoder. In this paper, we propose NVC-Net, an end-to-end adversarial network, which performs voice conversion directly on the raw audio waveform of arbitrary length. By disentangling the speaker identity from the speech content, NVC-Net is able to perform non-parallel traditional many-to-many voice conversion as well as zero-shot voice conversion from a short utterance of an unseen target speaker. Importantly, NVC-Net is non-autoregressive and fully convolutional, achieving fast inference. Our model is capable of producing samples at a rate of more than 3600 kHz on an NVIDIA V100 GPU, being orders of magnitude faster than state-of-the-art methods under the same hardware configurations. Objective and subjective evaluations on non-parallel many-to-many voice conversion tasks show that NVC-Net obtains competitive results with significantly fewer parameters.
Publication 13 Distributed Graph Learning with Smooth Data Priors
- Authors
- Isabela Cunha Maia Nobre, Mireille El Gheche, Pascal Frossard
- Abstract
- Graph learning is often a necessary step in processing or representing structured data, when the underlying graph is not given explicitly. Graph learning is generally performed centrally with a full knowledge of the graph signals, namely the data that lives on the graph nodes. However, there are settings where data cannot be collected easily or only with a non-negligible communication cost. In such cases, distributed processing appears as a natural solution, where the data stays mostly local and all processing is performed among neighbours nodes on the communication graph. We propose here a novel distributed graph learning algorithm, which permits to infer a graph from signal observations on the nodes under the assumption that the data is smooth on the target graph. We solve a distributed optimization problem with local projection constraints to infer a valid graph while limiting the communication costs. Our results show that the distributed approach has a lower communication cost than a centralised algorithm without compromising the accuracy in the inferred graph. It also scales better in communication costs with the increase of the network size, especially for sparse networks.
Publication 14 Heterogeneous Graph Node Classification with Multi-Hops Relation Features
- Authors
- Xiaolong Xu, Lingjuan Lyu, Hong Jin, Weiqiang Wang, Shuo Jia
- Abstract
- In recent years, knowledge graph~(KG) has obtained many achievements in both research and industrial fields. However, most KG algorithms consider node embedding with only structure and node features, but not relation features. In this paper, we propose a novel Heterogeneous Attention~(HAT) algorithm and use both node-based and path-based attention mechanisms to learn various types of nodes and edges on the KG. To better capture representations, multi-hop relation features are involved to generate edge embeddings and help the model obtain more semantic information. To capture a more complex representation, we design different encoder parameters for different types of nodes and edges in HAT. Extensive experiments validate that our HAT significantly outperforms the state-of-the-art methods on both the public datasets and a large-scale real-world fintech dataset.