- July 23~29, 2022
- Vienna, Austria
- ML/General AI
IJCAI 2022
IJCAI 2022, the 31st International Joint Conference on Artificial Intelligence
IJCAI has been a premier international gathering of AI researchers and practitioners since 1969. IJCAI-22 will be held in Vienna, Austria from July 23rd until July 29th . Sony will exhibit and participate as a Silver sponsor.
Recruiting information for IJCAI-2022
We look forward to highly motivated individuals applying to Sony so that we can work together to fill the world with emotion and pioneer the future with dreams and curiosity. Join us and be part of a diverse, innovative, creative, and original team to inspire the world.
For Sony AI positions, please see https://ai.sony/joinus/jobroles/.
*The special job offer for IJCAI-2022 has closed. Thank you for many applications.
Keynote Speech
Date & Time; July 26 (Tuesday) 14:00-15:00pm Training the world's best Gran Turismo racer
Automobile racing represents an extreme example of real-time decision making in complex physical environments. Drivers must execute complex tactical maneuvers to pass or block opponents while operating their vehicles at their traction limits. Modern racing simulations, such as the PlayStation game Gran Turismo, faithfully reproduce much of the nonlinear control challenges of real race cars while also encapsulating the complex multi-agent interactions. In this talk I will describe how our team at Sony AI trained agents for Gran Turismo that can compete with the world's best e-sports drivers. We combine state-of-the-art model-free deep reinforcement learning algorithms with mixed scenario training to learn an integrated control policy that combines exceptional speed with impressive tactics. In addition, we construct a reward function that enables the agent to be competitive while adhering to racing's important, but under-specified, sportsmanship rules. We demonstrate the capabilities of our agent, Gran Turismo Sophy, by winning a head-to-head competition against four of the world's best Gran Turismo drivers.
Technologies & Business use case
Technology 01 Enhancing games with cutting-edge AI to unlock new possibilities for game developers and players.
We are evolving Game-AI beyond rule-based systems by using deep reinforcement learning to train robust and challenging AI agents in gaming ecosystems. This technology enables game developers to design and deliver richer experiences for players. The recent demonstration of Gran Turismo Sophy™, a trained AI that beat world champions in the PlayStation™ game Gran Turismo™ SPORT, embodies the excitement and possibilities that emerge when modern AI is deployed in a rich gaming environment. As AI technology continues to evolve and mature, we believe it will help spark the imagination and creativity of game designers and players alike.
Can an AI outrace the best human Gran Turismo drivers in the world? Meet Gran Turismo Sophy and find out how the teams at Sony AI, Polyphony Digital Inc., and Sony Interactive Entertainment worked together to create this breakthrough technology. Gran Turismo Sophy is a groundbreaking achievement for AI, but there's more: it demonstrates the power of AI to deliver new gaming and entertainment experiences.
Technology 03 Character conversation AI technology
People wish to talk freely with their favorite characters, create AI characters on their own and provide various services using AI characters. At the Sony Group R&D Center, we are working on the character conversation AI technology to make these dreams come true. Interacting with characters in a more realistic way requires not just natural language processing technology for language understanding and generation but many other underlying technologies as well, including voice signal processing technology for voice recognition and speech synthesis and image processing technology for image recognition and graphic expression.
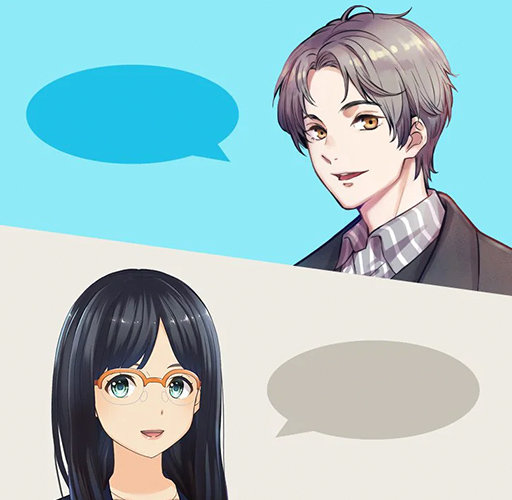
Technology 04 3D Environment Sensing
3D Environment Sensing is a technology which analyzes photos taken by a device to make it aware of its environment and estimate its position, then recreates a realistic 3D model of its surroundings. Applications include a wide range of fields, such as the entertainment, robotics, and video production industries.
Technology 05 Earth MIMAMORI platform
It all started from a simple idea, "Could we develop a system that enables sensing anywhere on Earth ?" Detecting signs of anomalies and issuing alerts, the system urges humanity to act with sustainability in mind, providing opportunities to think about what can be done to realize a sustainable future and take concrete action. We call this concept "MIMAMORI", which means to "watch over" in Japanese.
Publications
Publication 01 Dynamic Sparse Training for Deep Reinforcement Learning
- Authors
- Ghada Sokar, Elena Mocanu, Decebal Constantin Mocanu, Mykola Pechenizkiy, and Peter Stone
- Abstract
- Deep reinforcement learning (DRL) agents are trained through trial-and-error interactions with the environment. This leads to a long training time for dense neural networks to achieve good performance. Hence, prohibitive computation and memory resources are consumed. Recently, learning efficient DRL agents has received increasing attention. Yet, current methods focus on accelerating inference time. In this paper, we introduce for the first time a dynamic sparse training approach for deep reinforcement learning to accelerate the training process. The proposed approach trains a sparse neural network from scratch and dynamically adapts its topology to the changing data distribution during training. Experiments on continuous control tasks show that our dynamic sparse agents achieve higher performance than the equivalent dense methods, reduce the parameter count and floating-point operations (FLOPs) by 50%, and have a faster learning speed that enables reaching the performance of dense agents with 40-50% reduction in the training steps.
Publication 02 Data- Free Adversarial Knowledge Distillation for Graph Neural Networks
- Authors
- Yuanxin Zhuang, Lingjuan Lyu, Chuan Shi, Carl Yang, Lichao Sun
- Abstract
- Graph neural networks (GNNs) have been widely used in modeling graph structured data, owing to its impressive performance in a wide range of practical applications. Recently, knowledge distillation (KD) for GNNs has enabled remarkable progress in graph model compression and knowledge transfer. However, most of the existing KD methods require a large volume of real data, which are not readily available in practice, and may preclude their applicability in scenarios where the teacher model is trained on rare or hard to acquire datasets. To address this problem, we propose the first end-to-end framework for data-free adversarial knowledge distillation on graph structured data (DFAD-GNN). To be specific, our DFAD-GNN employs a generative adversarial network, which mainly consists of three components: a pre-trained teacher model and a student model are regarded as two discriminators, and a generator is utilized for deriving training graphs to distill knowledge from the teacher model into the student model. Extensive experiments on various benchmark models and six representative datasets demonstrate that our DFAD-GNN significantly surpasses state-of-the-art data-free baselines in the graph classification task.
Publication 03 Vertically Federated Graph Neural Network for Privacy-Preserving Node Classification
- Authors
- Chaochao Chen, Longfei Zheng, Huiwen Wu, Lingjuan Lyu, Jun Zhou, Jia Wu, Bingzhe Wu, Ziqi Liu, Li Wang, Xiaolin Zheng
- Abstract
- Graph Neural Network (GNN) has achieved remarkable progresses in various real-world tasks on graph data. High-performance GNN models always depend on both rich features and complete edge information in graph. However, such information could possibly be isolated by different data holders in practice, which is the so-called data isolation problem. To solve this problem, in this paper, we propose Vertically Federated Graph Neural Network (VFGNN), a federated GNN learning paradigm for privacy-preserving node classification task under data vertically partitioned setting, which can be generalized to existing GNN models. Specifically, we split the computation graph into two parts. We leave the private data (i.e., features, edges, and labels) related computations on data holders, and delegate the rest of computations to a semi-honest server. We also propose to apply differential privacy to prevent potential information leakage from the server. We conduct experiments on three benchmarks and the results demonstrate the effectiveness of VFGNN.
Other Conferences
-
- June 19~24, 2022
- New Orleans, Louisiana
- Computer Vision
CVPR 2022
IEEE Conference on Computer Vision and Pattern Recognition (CVPR) 2022
View our activities -
- May 7 ~ 13, 2022 /May 22 ~ 27, 2022
- Online / Singapore, China
- Signal Processing
ICASSP2022
2022 IEEE International Conference on Acoustics, Speech and Signal Processing
View our activities